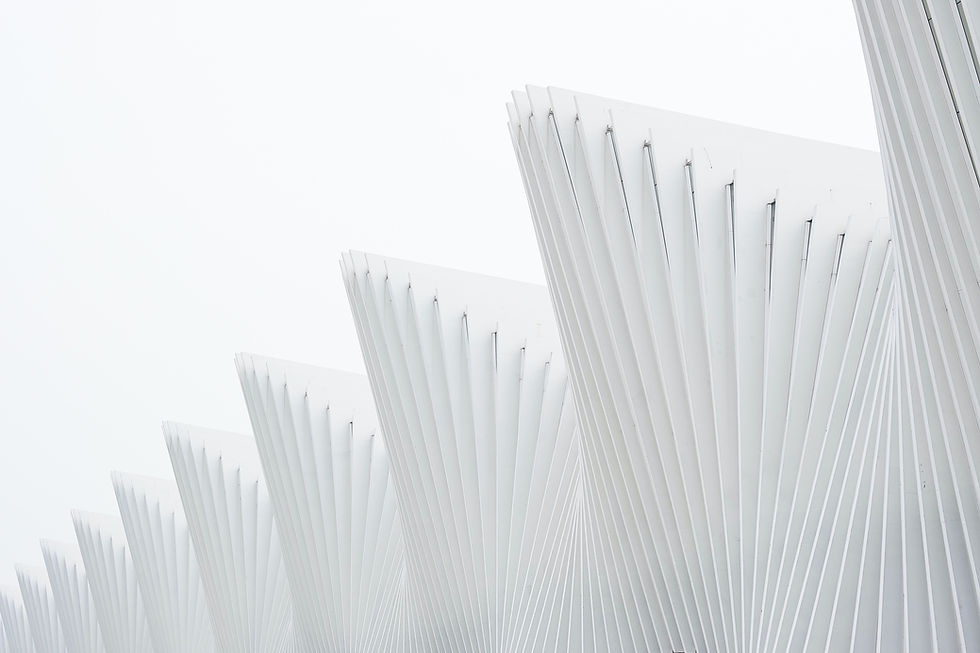
​
The Institute of Mathematical Statistics (IMS) is proud to introduce the annual IMS Frontiers in Statistical Machine Learning (FSML) workshop series. This series is dedicated to exploring emerging and impactful topics in the field of statistical machine learning that have yet to receive significant attention in leading IMS and ASA publications. Each year, the FSML workshop will spotlight 2-3 themes where research is rapidly evolving, encouraging the dissemination of novel ideas and fostering deeper engagement within the community.
Inspired by the dynamic format of machine learning conference workshops, FSML brings a fresh and interactive approach to the statistics landscape. The workshop will host an open call for short paper submissions, followed by a rigorous and transparent review process to ensure the highest quality of contributions. This inclusive model is designed to promote the exchange of innovative research, stimulating conversation and collaboration among attendees.
​
Accepted submissions will be presented through poster sessions and enriched by discussions that provide real-time feedback and networking opportunities. This structure is aimed at enhancing dialogue between researchers and practitioners, paving the way for future advances in the field.​​
The Inaugural Workshop on
Frontiers in Statistical Machine Learning (FSML)
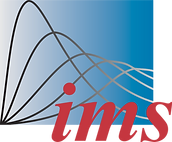
Conference Venue
​The inaugural FSML Workshop will be held on August 2, 2025, at Vanderbilt University in Nashville, Tennessee—immediately preceding the 2025 Joint Statistical Meetings (JSM) and following the 2025 IMS NRC meeting.
The program will take place in the Commodore Ballroom of Vanderbilt’s Student Life Center, a convenient 10-minute walk from the NRC’s Holiday Inn Nashville-Vanderbilt venue and about a 10-minute drive (or 30-minute bus ride) from the JSM site at the Music City Center.
Topics
There will be two main streams in the 2025 workshop:
​
1) The Science of Deep Learning
-
Theoretical Foundations: Exploring mathematical and statistical principles underlying deep learning.
-
Phenomenological Studies of Learning Systems: Cataloging and explaining intriguing behaviors in learning dynamics.
-
Interpretability, Alignment, and Safety: Understanding and guiding AI systems to ensure ethical and safe operation.
-
Emerging Learning Paradigms: Investigating new approaches, such as in-context learning and scaling laws.
-
Other Related Topics
​
2) Statistical Learning from Heterogeneous Data Sources and Generalization
-
Learning under Distribution Shifts: Addressing challenges caused by mismatches between training and test data, including covariate shifts, label shifts, and other distributional changes.
-
Distribution Shifts in Scientific Replications: Investigating how distributional changes impact the reproducibility and generalizability of scientific findings.
-
Domain Adaptation and Domain Generalization: Designing methods to adapt models to new domains or ensure robust performance across diverse domains.
-
Distributional Robustness: Developing techniques to maintain reliable performance under adversarial or worst-case distributional scenarios.
-
Semi-Supervised Learning: Combining labeled and unlabeled data to improve learning and generalization across heterogeneous sources, particularly when labeled data is scarce or distributions shift between domains.
-
Foundation Model Fine-Tuning: Leveraging pre-trained models for specific tasks or domains through transfer learning and fine-tuning.
-
Other Related Topics
Invited Speakers
Meet our distinguished team of young researchers and academics who will be sharing their insights at the FSML on 1) the science of deep learning and 2) statistical learning from heterogeneous data sources and generalization. Each invited speaker brings a unique perspective and expertise to the workshop.
Session One: The Science of Deep Learning

Qi Lei
New York University
Talk Title: TBA
Talk Abstract: TBA

Samet Oymak
University of Michigan
Talk Title: TBA
Talk Abstract: TBA
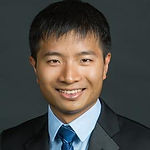
Weijie Su
University of Pennsylvania
Talk Title: TBA
Talk Abstract: TBA

Danica J. Sutherland
University of British Columbia
Talk Title: TBA
Talk Abstract: TBA
Session Two: Statistical learning from heterogeneous data sources and generalization
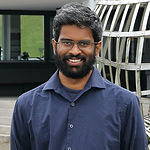
Sivaraman Balakrishnan
Carnegie Mellon
Talk Title: TBA
Talk Abstract: TBA
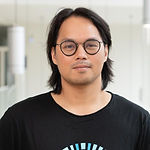
Krikamol Muandet
CISPA Helmholtz Center for Information Security
Talk Title: TBA
Talk Abstract: TBA

Dominik Rothenhausler
Stanford University
Talk Title: TBA
Talk Abstract: TBA

Xinwei Shen
University of Washington
Talk Title: TBA
Talk Abstract: TBA
Schedule
Session 1: Science of Deep Learning
-
9:30-10:05​​: Invited Talk 1
-
10:05-10:40: Invited Talk 2
-
10:40-11:00: Break
-
11:00-11:35: Invited Talk 3
-
11:35-12:10: Invited Talk 4
1210-1400: Lunch
Session 2: Statistical Learning from Heterogeneous Data Sources
-
14:00-14:35​​: Invited Talk 1
-
14:35-15:10: Invited Talk 2
-
15:10-15:30: Break
-
15:30-16:05: Invited Talk 3
-
16:05-16:40: Invited Talk 4
​​
17:00-18:45: Poster session
Important Dates
​See the important dates below:​
-
Paper submission Round 1: March 2, 2025 (11:59 PM AoE)
-
Paper submission Round 2: April 2, 2025 (11:59 PM AoE)
-
Notice of acceptance: May 2, 2025
-
Registration deadline: July 2nd, 2025 (same as the end of JSM regular registration deadline)
-
Conference: All day, 2 August, 2025​​
Submission Details
Paper Submission:
-
The review process for the workshop will be single-blind, i.e. authors will not know the reviewers' identity.
-
Submissions can be existing work or be currently under review at another venue.
-
This workshop does not host archival proceedings. Authors are encouraged to confirm dual submission policies with journals or conferences. For instance, submitting to both this workshop and NeurIPS typically does not violate their dual submission policy.
-
Each paper is limited to 5 pages, not including references and supplementary material.
-
Please use the TMLR Latex Style and Template, which can be found in https://jmlr.org/tmlr/author-guide.html.
-
If you encounter any problems with the submission of your papers, please contact the organizers' group email.​​
-
The workshop will adopt OpenReview as a submission system (click here for the submission portal), which will open February 1st, 2025.
​
Travel awards:
The IMS is pleased to offer $500USD travel awards to support participation in the workshop. The top 10 applicants, determined based on the quality of their paper submissions, will receive these awards.
​
-
Application Process:
-
Travel award applications must be submitted alongside your paper submission on OpenReview. Please ensure you check the designated box during the submission process to indicate your interest in applying for the travel award.
-
Along with your application, you are required to upload a CV through the OpenReview system.
-
​
-
​Eligibility: If you have already applied for any of the following three IMS travel awards this year, you are not eligible for our travel award:
-
IMS Hannan Graduate Student Travel Award
-
IMS New Researcher Travel Award
-
NRC Conference Travel Cost Reimbursement (details available at NRC2024).
-
Post-submission updates:
After a competitive review process, we awarded ten travel grants to the best papers with lead student authors. They are (in no particular order):
-
Heterogeneous transfer learning for high dimensional regression with feature mismatch
Jae Ho Chang, Subhadeep Paul, Massimiliano Russo -
Out-of-distribution generalization via composition: a lens through induction heads in Transformers
Jiajun Song, Zhuoyan Xu, Yiqiao Zhong -
Double Descent and Overfitting under Noisy Inputs and Distribution Shift for Linear Denoisers
Chinmaya Kausik, Kashvi Srivastava, Rishi Sonthalia -
A Statistical Theory of Overfitting for Imbalanced Classification in Deep Learning
Jingyang Lyu, Kangjie Zhou, Yiqiao Zhong -
Detecting Topological Changes and OOD Misplacement in t-SNE and UMAP via Leave-one-out
Zhexuan Liu, Rong Ma, Yiqiao Zhong -
A Statistical Theory of Contrastive Learning via Approximate Sufficient Statistics
Licong Lin, Song Mei -
Minimax And Adaptive Transfer Learning for Nonparametric Classification under Distributed Differential Privacy Constraints
Arnab Auddy, T. Tony Cai, Abhinav Chakraborty -
Transfer Learning for Survival-based Clustering of Predictors with an Application to TP53 Mutation Annotation
Xiaoqian Liu, Hao Yan, Haoming Shi, Emilie Montellier, Eric Chi, Pierre Hainaut, Wenyi Wang -
Task Shift: From Classification to Regression in Overparameterized Linear Models
Tyler LaBonte, Kuo-Wei Lai, Vidya Muthukumar -
Active-Dormant Attention Heads: Mechanistically Demystifying Extreme-Token Phenomena in LLMs
Tianyu Guo, Druv Pai, Yu Bai, Jiantao Jiao, Michael I. Jordan, Song Mei
​
​​
Poster Instructions
Portrait orientation: horizontal
Maximum dimensions: 91 inches × 45 inches (231 cm × 114 cm)
Your poster will be displayed on white foamboard standee size 94 inches × 48 inches (200 cm × 100 cm) portrait. We will provide push pins for installing the posters.
Registration
There are three types of registration, including a special offer for students to attend our workshop:​
-
IMS Member - US$150
-
Non-IMS Member - US$175
-
Student - US$75​
Please click here to register.
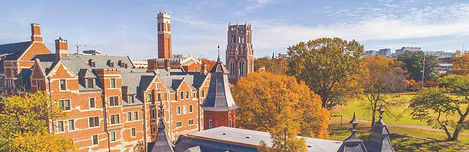
ORGANISERS (alphabetical order)
Program Committee
Local Committee
GET IN TOUCH
If you have questions about the submission/registration process, don’t hesitate to reach out.
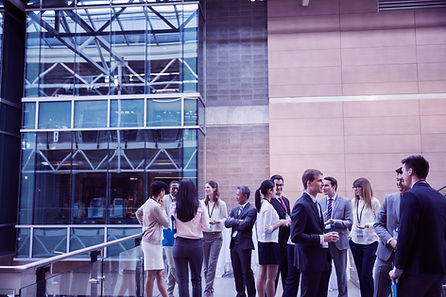